Visual-Pattern Detector (VPD)
Automatic pattern detection has become increasingly important for scholars in the Humanities, since their research benefits more and more from the growing amount of digitised manuscripts. Nevertheless, most of the state-of-the-art methods for pattern detection depend on the availability of a large number of training samples, which are typically not available in the Humanities as they involve tedious manual annotations by the scholars (e.g. marking location and size of words, drawings, seals, etc.). Furthermore, scholars often deal with a small number of images within the scope of a specific research question. Even if a large number of images are available, most of the manuscripts that contain them do not contain any ground-truth information, such as related metadata or transcriptions. This makes the applicability of such methods very limited within the field of manuscript research.
Therefore, we developed a state-of-the-art training-free pattern detection method for the task of detecting patterns such as drawings, seals, words, and letters in images of written artefacts without the need of any training data.
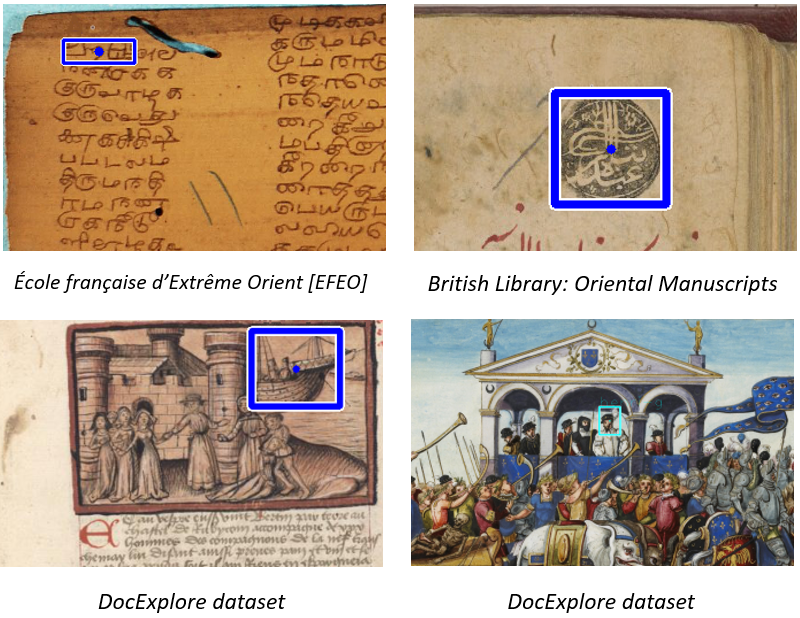
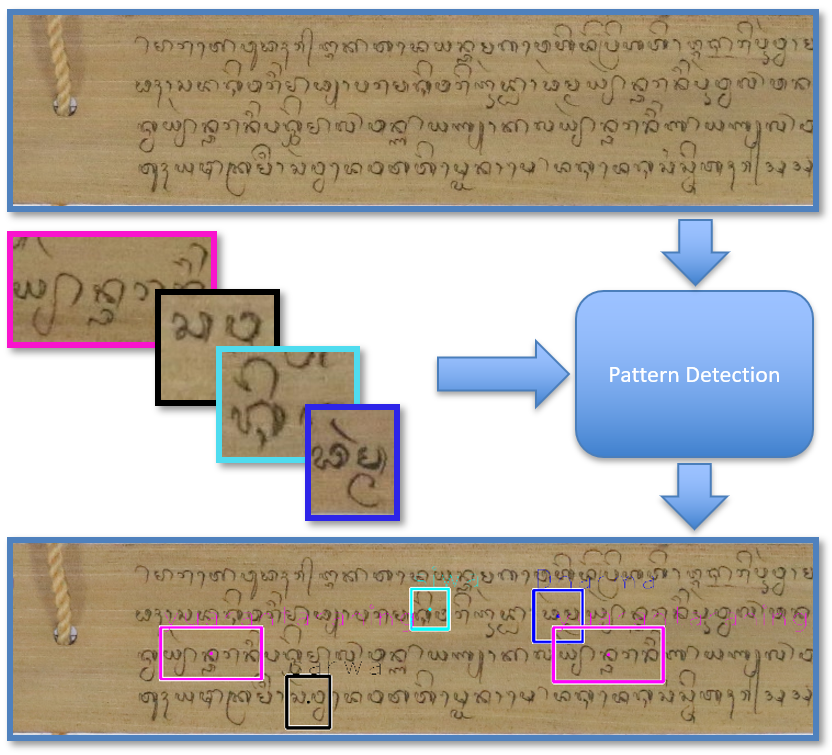
Our developed method can be used to search for several patterns in a large number of images concurrently using the computational resources offered by our local server. Furthermore, a software tool has been developed to offer the possibility of smaller searches in any personal computer.
VPD is an efficient and easy-to-use software tool for pattern detection, which is based on the proposed method in "Learning-free pattern detection for manuscript research". This tool can be used to recognise and allocate visual patterns (such as words, drawings and seals) automatically in digitised manuscripts. The recall-precision balance of detected patterns can be controlled visually, and the detected patterns can be saved as annotations on the original images or as cropped images depending on the needs of users. More details can be found here.
Example of letter detection using VPD. Detection threshold can be controlled visually.
As for the cases where few annotated examples are available, a state-of-the-art, training-based approach has been developed. This approach is capable of detecting very small visual patterns in manuscript images using very few training examples. The computational resources required by this approach are already available in our lab.